Is Big Data Useful For The Fleet Industry?
Making use of big data for improving efficiency is the watch word that the fleet industry has been hearing about for quite some time now.
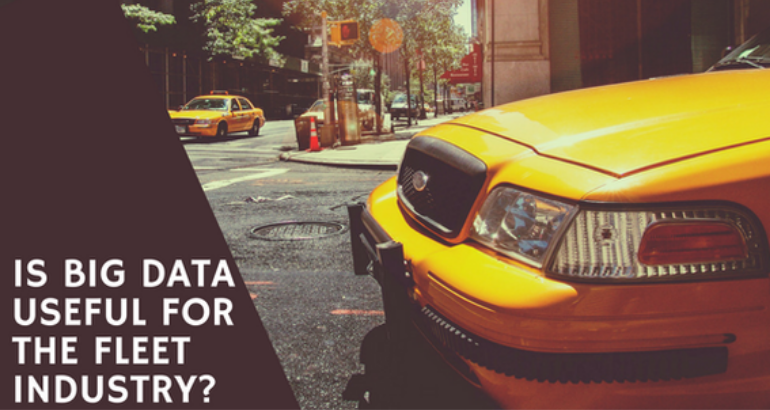
Is Big Data useful for the fleet industry?
Making use of big data for improving efficiency is the watch word that the fleet industry has been hearing about for quite some time now. What are the areas in the fleet industry that big data has actually impacted? Routing and navigation is one such field where people claim to have found the maximum impact, followed by supply chain management. But is this claim of impact actually true? A mid-sized telematics company servicing around ten thousand vehicles will still not have the critical mass to do routing and navigation efficiently since it would need millions of vehicles. As a result, companies have to use core APIs from mapping companies like Google and HERE and provide value added services over it. In case of supply chain management, the barriers of sharing data between different companies in the supply chain has resulted in this being impactful for only large corporations servicing different parts of the supply chain. Is the pursuit of big data then futile for the fleet industry?
When it comes to deriving benefits through insights from data, video data, or visual analytics, offers the most tangible value without the need for colossal amounts of data. There is no need for a huge critical mass of vehicles to be continuously supplying data for this to create an impact. Insights can be obtained at the driver level, fleet level and across fleets. Driver behaviour and safety is the first level of analytics that encompasses solutions like forward collision warning, lane keeping, speed limit compliance etc. Fleet level analytics will bring to the fore parameters of efficiency, such as fuel savings and maintenance costs. These parameters will have a direct relation with driver behaviour. From aggregating driver level analytics, the overall health of the fleet in terms of driving patterns will emerge, which can be directly translated to the key performance indicators for example, more following distance violation events across the fleet will indicate not only a higher fuel consumption but also a greater risk of a rear ending incident. The next scale would be aggregating data across fleets to give much more advanced video analytics, e.g. advanced machine learning algorithms which can predict accidents from video data based on past history and warn the driver before the accident happens.
In conclusion, obtaining insights from video data would be much more actionable, the fruits of which can be reaped without having to reach a much higher critical mass of vehicles.