Video Telematics with AI on the Edge – What, Why and How
Cameras are fast becoming ubiquitous in commercial fleets, especially from a driver exoneration point of view.
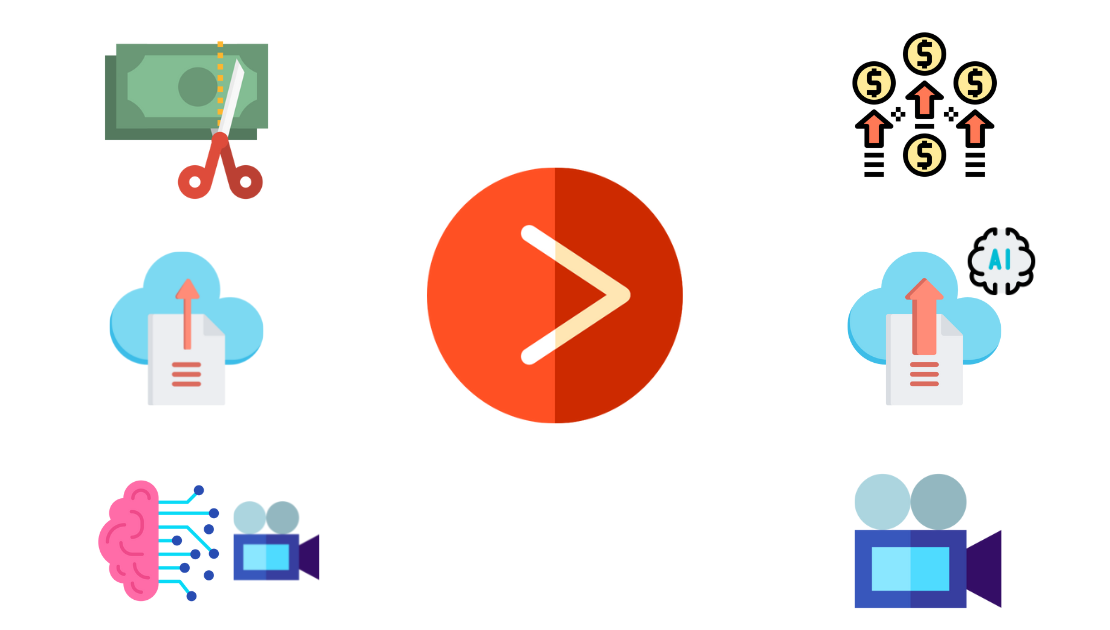
What is AI on the Edge?
Cameras are fast becoming ubiquitous in commercial fleets, especially from a driver exoneration point of view. With all the recent advances in AI (artificial intelligence) and ML (machine learning), however, the benefits are no longer limited to just exoneration. They include:
- Accident prevention – real-time notifications for events like drowsiness and tailgating, that serve as a driver alert.
- Real-time coaching – alerts for stop sign violation, speeding, distraction, etc. that act like a virtual coach sitting in the cabin.
- Triggers beyond braking and acceleration – coachable events with video snippets based on triggers that are more causal indicators of risk, such as distracted driving.
- Positive behavior recognition – events like hard braking when cut off by a vehicle, reducing speed when notified of a posted speed limit, increasing headway when receiving the following distance warning, etc.
- Mapping drivers to trips using face recognition – achieve compliance with regulations like ELD/HOS in a near painless manner.
- Efficient fleet manager workflows – actionable insights in terms of identifying safest drivers, drivers who need coaching, areas drivers need to be coached in, auto-curation of the most severe event videos that can be used for coaching, etc.
It is clear from many of the use-cases above that the analysis of video and other sensory data has to happen in real-time, to be able to provide the benefits that matter. Real-time analysis implies that video has to be analyzed locally (AI on the Edge) – it cannot be uploaded to the cloud for analysis in a data center and have the insights still be delivered on time. There is also the not insignificant cost of uploading streaming video content. Additionally, computing on the cloud is an expensive resource, and processing videos from even thousands of vehicles on a public cloud service can add significantly to the overall cost of a video telematics solution. These costs can be avoided completely with the local analysis of video and other data, the delivery of applicable insights in real-time, and the upload of metadata and event videos to the cloud to enable post-hoc insights for the fleet manager and drivers.
In a nutshell, AI on the Edge is the fast and efficient delivery of insights, almost completely using the computing resources available locally on device.
Why do fleets need it?
Safety in commercial vehicles is a business-critical function because it has a near-direct impact on the business viability of fleets. Done right, fleets can have fewer accidents, lesser damages, lower maintenance, better mileage, driver retention and engagement, lower insurance rates, and much more – the RoI equation is heavily loaded in favor of massive savings.
Accident prevention due to real-time driver feedback is a cut and dried scenario from a fleets point of view – the RoI is immediate and tangible. Going further, deeper, and more actionable insights into fleet safety have also traditionally been a very high-value service in the telematics industry. Before the advent of modern ML and AI, this was delivered through bureau services. Triggers from conventional telematics, including, but not limited to, hard braking, hard cornering, etc. were used to capture video snippets, and trained human agents would score these event videos. For example, if there was a harsh braking event, the human observer would annotate if the driver was also distracted by looking at her phone. In this way, using basic triggers, the second level of insights were added on and delivered in a highly actionable form to the fleet, leading to effective driver behavior change. On the flip side, this meant more data usage, delays due to manual analysis, and a significantly more expensive offering due to the involvement of bureau services.
With AI on the Edge, there is now the real possibility that fleets can obtain deeper insights in a more scalable, automated, and cost-efficient manner. While AI-based analysis can possibly replace bureau services in terms of achieving objectives of a fleet with respect to KPIs, they can also be looked upon as making bureau services extremely efficient. If TSPs or fleets want to run managed coaching services, AI and ML can make the job of the bureau so much easier.
How is it done?
Deep neural networks used in today’s AI and ML-based solutions are complex in many ways. They are usually big (more memory), computationally complex (need specialized processing hardware like ASICs and GPUs), and need lots of annotated data for training them in the first place. From a fleet’s perspective, this means that solutions having AI on the Edge are likely to be expensive – especially from a hardware perspective.
At LightMetrics, one of our USPs is being able to do AI on commodity hardware, including on ARM processors. For our partners, this leads to a broader choice of hardware when it comes to providing fleets and drivers with cutting-edge benefits. For fleets, this means a better return on investment. From an ecosystem point of view, this is a true win-win situation for everyone.
There are two key areas that we focus on at LightMetrics, as it relates to efficient AI:
- Efficient inference
- Efficient training
Inference refers to the process of analyzing data (video or image in this instance) generated in everyday operation, typically with a neural net. It does not matter how complex training is since it can run offline, or in data centers which have sophisticated GPUs and almost unlimited computing capacity available. Inference, on the other hand, has to be extremely efficient, especially if done on the edge. By efficiency, we refer to factors such as memory and computations. It has to be noted that these two factors have further ramifications in terms of the chipset used, heat dissipation and power consumption – all of which drive the cost of hardware up.
The way we make neural nets efficient is by pruning. Pruning refers to the process of determining the actual importance of every neuron and every channel in a neural network towards the end result and removing what can be removed. Neural nets are heavily over-parameterized, which means one can prune it quite well. How to prune, what channels to remove, what neurons to retain, are cutting-edge areas of research in neural networks today.
Efficient training refers to efficiency in terms of the amount of raw data needed, amount of annotated data (data that has gone through manual analysis), and the quality of the data annotations. With a lot of high quality annotated data, the task of training neural networks becomes easier. However, high-quality annotations are very expensive. Further, for many problems, high amounts of data are not always readily available. To have a quick turnaround for new features that deliver new benefits, efficient training is a must. This involves exploring neural net architectures that are known to be efficient in learning, semi-supervised learning, unsupervised learning, and active learning. Semi-supervised and unsupervised learning are able to ingest large amounts of unannotated data or data that does not have extremely high-quality annotations. Active learning helps us focus on the expensive exercise of high-quality labeling or annotation on the data that really matters.
With efficient training and inference, we are able to deliver world-class solutions using AI on the Edge, that does not need overly specialized hardware, helping us deliver outsized benefits on reasonably priced hardware. This ensures the best return on investment for our partners as well as end-user fleets. With cameras becoming more accepted by drivers, and fleets having a better understanding of the benefits of AI, there has never been a more exciting team to be creating new solutions and providing value to drivers and the fleets they work for.
Combining the core competence of AI on the Edge with the knowledge of how video telematics should be architected to provide the highest value to the end-user, LightMetrics is in a unique position to help our partners do more using AI – capturing more value (ARPU) and providing even greater value. Talk to us to learn more, and lead from the front in the most exciting space in fleet telematics.